Automation: A Game Changer in EHR Optimization
- Sai Sravan Cherukuri
- Mar 7
- 4 min read
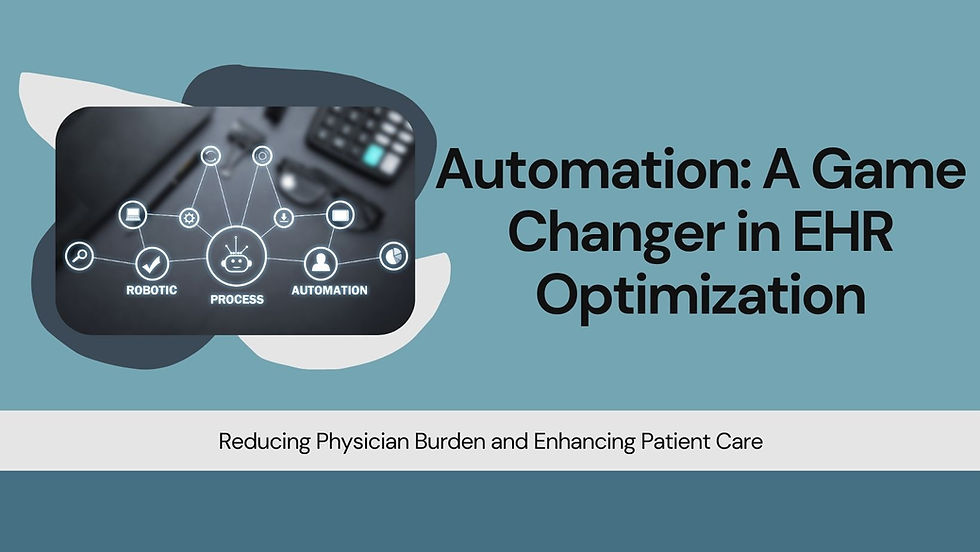
The rapid adoption of Electronic Health Records (EHRs) was intended to streamline patient information management and improve care delivery. However, the unintended consequence has been a significant administrative burden on physicians, leading to burnout, decreased efficiency, and compromised patient interactions. This whitepaper explores how automation, through Artificial Intelligence (AI) and Natural Language Processing (NLP), can alleviate the documentation burden, enhance interoperability, and enable clinicians to focus on patient care.
Introduction
Physicians today spend an inordinate amount of time on documentation due to stringent regulatory requirements and the complexity of EHR systems. Instead of simplifying workflows, traditional EHR systems have created inefficiencies that take away valuable time from direct patient care. The solution is leveraging automation to optimize documentation processes, minimize manual data entry, and facilitate seamless clinical workflows.
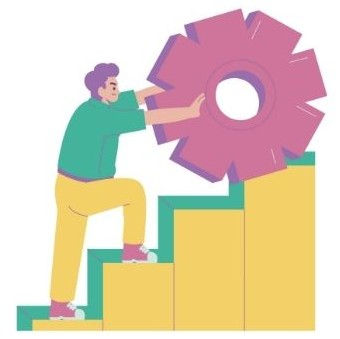
Challenges with Current EHR Systems
Excessive Documentation Load – Physicians spend nearly 50% of their work hours on EHR-related tasks, often after clinic hours (so-called "pajama time").
Burnout & Physician Dissatisfaction – Administrative overhead contributes to stress, exhaustion, and decreased job satisfaction.
Data Entry Errors & Redundancies – Manual inputs lead to mistakes and inconsistencies, impacting patient safety.
Lack of Interoperability – Healthcare data silos hinder care coordination and continuity.

Automation: A Game Changer in EHR Optimization
Automation technologies such as AI, NLP, and robotic process automation (RPA) present an opportunity to transform EHR functionality. The following automation strategies can significantly reduce physician workload:
1. AI-Driven Voice Recognition & NLP for Clinical Documentation
AI-powered transcription tools can convert physician speech into structured notes in real-time.
NLP-based algorithms extract key medical data from conversations, eliminating redundant typing.
Intelligent summarization automates patient history generation and chart updates.
2. Automated Data Capture & Entry
Integration with medical devices and lab systems to auto-populate records.
Optical character recognition (OCR) for scanning and extracting data from paper-based records.
AI-driven data validation to reduce manual corrections.
3. Predictive Analytics for Decision Support
Machine learning models analyze patient history to flag high-risk cases.
Automated alerts for potential drug interactions or abnormal test results.
AI-assisted recommendations based on population health data.
4. Enhanced Interoperability Through Automation
Standardized APIs to facilitate seamless data exchange between EHR platforms.
AI-driven data mapping to bridge discrepancies across different systems.
Blockchain-based verification for secure, interoperable patient data sharing.
5. Workflow Automation for Routine Administrative Tasks
Automated appointment scheduling and follow-ups.
Smart order sets and protocol-driven documentation.
Integrated referral and discharge processes.
Key Technologies Enabling EHR Automation
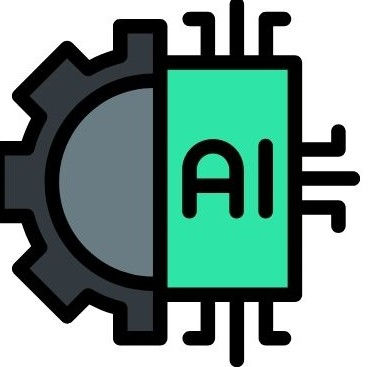
1. Artificial Intelligence (AI) & Machine Learning (ML)
AI and ML play a pivotal role in automating EHR documentation and decision support by enabling:
AI-powered Speech Recognition & Transcription: Converts physician speech into structured EHR notes (e.g., Nuance Dragon Medical One).
Clinical Note Summarization: AI extracts key patient data to generate concise documentation.
Predictive Analytics: ML models analyze patterns in patient data to identify potential health risks and suggest preventive measures.
2. Natural Language Processing (NLP)
NLP is essential for making EHRs more user-friendly by:
Extracting structured data from unstructured clinical notes.
Enhancing physician queries by understanding intent (e.g., "Show me last month's lab results").
Automating responses for patient inquiries in telemedicine.
3. Robotic Process Automation (RPA)
RPA automates routine and repetitive administrative tasks, including:
Data entry and record updates.
Billing and coding processes.
Interoperability workflows between different health systems.
4. Interoperability & API Integration
FHIR (Fast Healthcare Interoperability Resources): Ensures seamless data exchange between EHRs.
HL7 Standards: Facilitates structured communication between different systems.
Blockchain Technology: Strengthens security in patient data sharing across institutions.
5. Computer Vision & Optical Character Recognition (OCR)
Scans and digitizes handwritten notes, lab reports, and legacy documents.
Reduces reliance on manual input for older patient records.
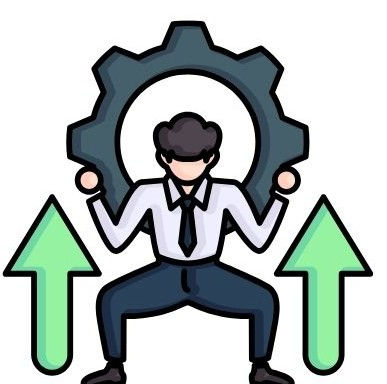
Implementation Challenges & How to Overcome Them
1. Physician Resistance to Change
Challenge: Clinicians may resist automation due to fears of losing control over documentation or concerns about AI accuracy.
Solution: Involve physicians in the design and testing phases; provide hands-on training with automation tools.
2. Data Privacy & Security Concerns
Challenge: EHR data contains sensitive patient information, and AI-driven automation must comply with HIPAA and GDPR regulations.
Solution: Implement end-to-end encryption, strict access controls, and blockchain-based security.
3. Integration with Legacy EHR Systems
Challenge: Many hospitals use outdated EHRs that lack API support for seamless automation.
Solution: Develop middleware solutions that bridge old EHRs with modern AI-powered automation tools.
4. Accuracy & Reliability of AI Models
Challenge: AI-driven NLP and voice recognition tools may misinterpret complex medical terminology.
Solution: Continuously train AI models on diverse datasets to improve accuracy and adapt to specialty-specific language.
5. Regulatory Compliance & Documentation Standards
Challenge: Automation must align with CMS, ONC, and other healthcare regulations.
Solution: Work with regulatory experts to ensure AI-based documentation meets legal and reimbursement guidelines.
Roadmap for Implementing EHR Automation

Phase 1: Assessment & Strategy Development (0-6 months)
Conduct workflow analysis to identify pain points in EHR usage.
Evaluate current EHR capabilities and integration possibilities.
Engage key stakeholders, including physicians, IT teams, and administrators.
Develop an automation strategy aligned with regulatory compliance.
Phase 2: AI & NLP Integration (6-12 months)
Pilot AI-driven speech-to-text solutions for real-time documentation.
Deploy NLP-based summarization for clinical note generation.
Train AI models on specialty-specific medical terminology.
Phase 3: Automation of Data Capture & Entry (12-18 months)
Implement direct EHR integration with lab systems and imaging tools.
Enable automated data extraction from structured and unstructured sources.
Establish automated error detection and validation mechanisms.
Phase 4: Predictive Analytics & Decision Support (18-24 months)
Deploy AI-powered risk stratification and predictive analytics.
Integrate decision-support tools within the EHR workflow.
Establish mechanisms for continuous model refinement.
Phase 5: Interoperability & Scalable Automation (24+ months)
Standardize data exchange protocols across health institutions.
Expand automation capabilities to additional administrative functions.
Continuously monitor the impact of physician feedback and refine solutions.
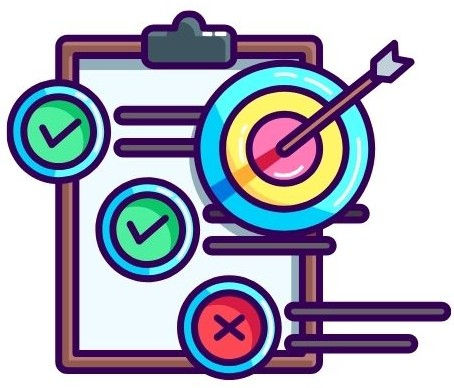
Expected Outcomes & Benefits
Reduced Physician Burnout – Less time on documentation, more focus on patient care.
Enhanced Accuracy & Compliance – AI-driven automation minimizes errors and meets regulatory requirements.
Improved Efficiency – Faster data entry, real-time note generation, and seamless interoperability.
Better Patient Outcomes – Predictive analytics aid in early diagnosis and treatment planning.
Cost Savings – Reduced administrative overhead leads to lower operational costs.
Conclusion
The integration of automation within EHRs is essential for transforming healthcare efficiency. AI-driven solutions can significantly reduce physician documentation burdens, allowing them to focus on patient care. Future advancements in automation will continue to enhance healthcare delivery, making it more efficient, intelligent, and patient-centric. A strategic, phased approach that combines technology adoption with physician engagement will be key to long-term success.